The Power of Predictive Analytics: Harnessing Data Science for Insights
"The Power of Predictive Analytics: Harnessing Data Science for Insights" delves into the transformative potential of predictive analytics in data science. Explore how predictive models anticipate future trends, optimize decision-making, and drive innovation across industries, empowering businesses to stay ahead in a data-driven world.
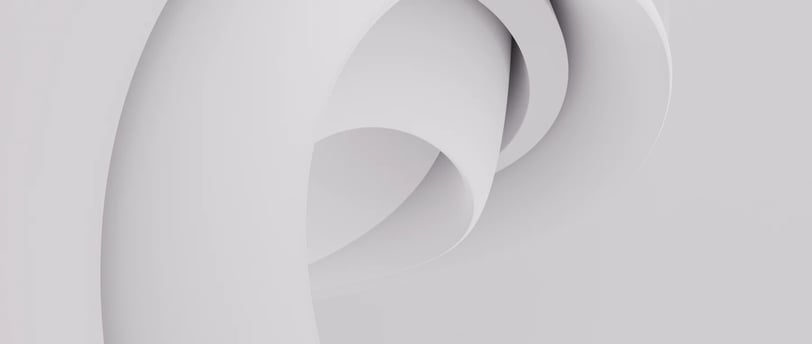
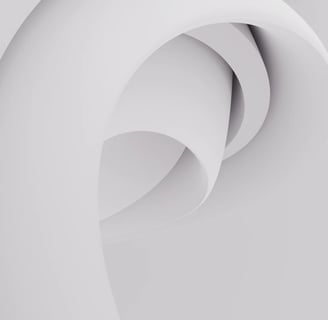
Businesses are using predictive analytics more and more in the data-driven age to stay competitive and obtain insightful information. Within the field of data science, predictive analytics forecasts future events by utilizing statistical algorithms and previous data. Businesses may predict trends, spot opportunities, and reduce risks by utilizing sophisticated modeling approaches, which will ultimately lead to better decision-making.
Understanding Predictive Analytics
Building predictive models that can foretell future occurrences or behaviors requires the application of past data in predictive analytics. To generate extremely accurate predictions, these models examine trends, correlations, and patterns in the data. Predictive analytics helps firms to drive strategic goals, enhance operations, and predict customer behavior by discovering correlations between factors and projecting future patterns.
Applications of Predictive Analytics
Predictive analytics has many different uses in a wide range of industries, including marketing, finance, healthcare, and manufacturing. Predictive analytics is used in marketing to optimize pricing tactics, segment customers, and personalize promotions. Predictive analytics is used in finance to manage investment portfolios, detect fraudulent transactions, and predict market trends. Predictive analytics helps with patient risk assessment, disease prediction, and therapy optimization in the healthcare industry. Predictive analytics in manufacturing increases production efficiency, forecasts equipment problems, and optimizes supply chain management.
Benefits of Predictive Analytics
Predictive analytics has many advantages. Businesses can obtain a competitive edge, make better decisions, and increase operational efficiency by utilizing data-driven insights. Businesses may foresee market trends, spot new possibilities, and reduce risks by utilizing predictive analytics. Through process and resource optimization, businesses can lower expenses, boost output, and stimulate revenue growth. In the end, predictive analytics gives companies the ability to make more strategic, astute decisions that spur growth and innovation.
Challenges of Predictive Analytics
Predictive analytics has enormous potential, but there are drawbacks as well. Data availability and quality is a major obstacle. For predictive models to produce precise forecasts, they need relevant, high-quality data. Predictions may be prejudiced or wrong as a result of poor data quality, inadequate data sets, and data biases. Interpretability and model complexity are another difficulty. Complex predictive models can be challenging to understand and interpret, which can cause suspicion or skepticism among stakeholders. Furthermore, while using predictive analytics solutions, ethical issues like privacy, justice, and openness need to be carefully taken into account.
Future Trends in Predictive Analytics
With developments in big data, machine learning, and artificial intelligence propelling innovation in the industry, predictive analytics has a bright future. Predictive analytics will become more widely available, scalable, and intuitive as technology advances, allowing companies of all kinds to take use of its potential. Predictive analytics will change in the future due to trends like explainable AI, automated machine learning, and real-time analytics, which will present new chances for companies to obtain valuable insights and create value.
Challenges in Implementation
Predictive analytics implementation presents a number of difficulties for businesses. The necessity for qualified data scientists and analysts who can create and implement predictive models successfully is one of the primary obstacles. It can be difficult to find and keep talent in this sector, especially considering how much demand there is for data science knowledge. In order to support predictive analytics programs, firms must also invest in infrastructure, tools, and technology, which can be expensive and time-consuming.
Data Quality and Governance
The accuracy and dependability of the underlying data are critical to the success of predictive analytics. Predictive models can lose accuracy and efficacy due to missing datasets, biased data, and poor quality data. Thus, in order to guarantee that the data utilized for predictive analytics is accurate, dependable, and representative of the underlying population, businesses need to give top priority to data quality assurance and governance procedures. To preserve the integrity of the data, this entails putting in place data quality checks, data cleansing procedures, and data governance frameworks.
Ethical Considerations
The use of predictive analytics brings up significant ethical questions about openness, justice, and privacy. Predictive models are becoming more and more important in decision-making processes related to finance, healthcare, and criminal justice; therefore, it is imperative to make sure these models are impartial, fair, and responsible. When implementing predictive analytics solutions, organizations need to handle ethical issues like prejudice, algorithmic bias, and unexpected repercussions. To achieve this, it is necessary to put ethical standards into place, evaluate prejudice, and promote accountability and openness in predictive modeling procedures.
Regulatory Compliance
Organizations using predictive analytics should also take regulatory compliance into account, especially in sectors with strict regulations like healthcare, banking, and telecommunications. Businesses need to make sure that their predictive analytics projects abide by all applicable laws, such as GDPR in the EU and HIPAA in the healthcare industry. This entails putting data protection mechanisms into place, getting the required permissions, and abiding by the ethical and legal guidelines for data use and privacy.
Continuous Improvement and Evaluation
Predictive analytics is a continual process of review and improvement rather than a one-time endeavor. To keep their prediction models accurate and useful, organizations must evaluate their predictive model performance on a regular basis, keep an eye out for modifications in data patterns or trends, and recalibrate the models as necessary. To enhance and improve predictive analytics systems over time, this entails setting up key performance indicators (KPIs), testing and validating models, and incorporating end-user feedback. Through the implementation of a data-centric strategy for ongoing enhancement, establishments can optimize the worth and influence of their predictive analytics endeavors.
Interpretability and Explainability
The demand for models to be comprehensible and elucidable is increasing with the sophistication of predictive analytics. Interpretable models facilitate stakeholder understanding of the prediction process and the rationale behind suggested decisions. Explainability is especially critical in regulated businesses where accountability and transparency are critical. To guarantee that decisions are credible and understood by stakeholders, organizations must strike a balance between the interpretability required of predictive models and their complexity.
Scalability and Integration
Another factor to take into account for businesses using predictive analytics is scalability. Organizations need to make sure that their predictive analytics infrastructure can manage massive datasets and complicated models as data volumes and computational needs rise. To meet the increasing needs of predictive analytics applications, this calls for investments in scalable computing resources, such as distributed computing frameworks and cloud-based infrastructure. To fully utilize predictive insights and provide meaningful results, firms must incorporate predictive analytics into their current business processes and systems.
Educating Stakeholders and Building Trust
Stakeholders from across the organization must support and buy into the effective use of predictive analytics. Building trust and acceptance requires educating stakeholders on the advantages, constraints, and ramifications of predictive analytics. To make sure that stakeholders understand how predictive analytics can help decision-making and produce value, organizations must invest in training programs, workshops, and communication initiatives. Transparency, accountability, and open communication regarding the data, models, and underlying assumptions of predictive insights are necessary to establish trust in predictive analytics. Organizations may harness the benefits of predictive analytics and promote positive business results by educating stakeholders and fostering trust.
Conclusion
To sum up, predictive analytics is an effective tool that helps companies uncover important information, foresee trends, and make more informed decisions. Businesses can improve operations, spur innovation, and obtain a competitive edge by utilizing data science. Predictive analytics will become more and more important in determining how business and society develop in the future as technology advances. Unlock the promise of data-driven insights by embracing the power of predictive analytics.