"Machine Learning in Practice: Realworld Applications and Case Studies"
Blog post description.
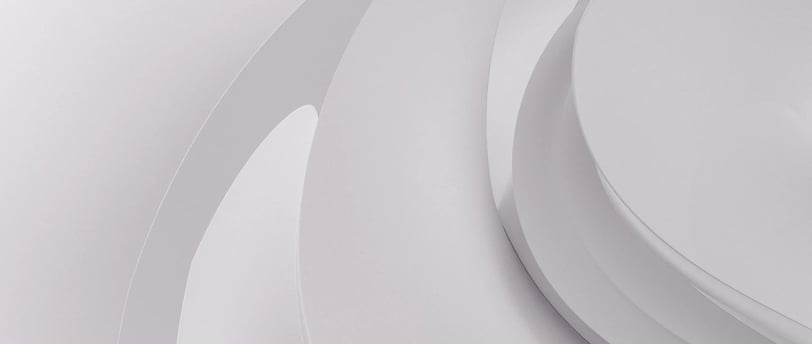
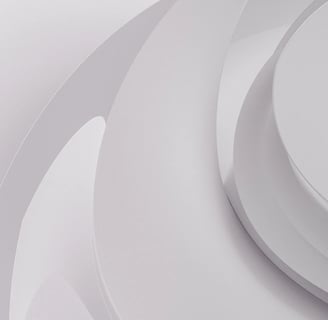
Introduction:
Outside of academic research, machine learning has become an essential part of many industries, such as banking, healthcare, and e-commerce. This blog post will look at real-world applications of machine learning as well as case studies that highlight its significance and impact. By examining the application of machine learning in a variety of fields, we hope to provide light on the benefits and challenges of implementing these techniques in real-world situations.
1. Healthcare:
Machine learning has developed into a powerful tool for enhancing patient outcomes, raising diagnostic accuracy, and streamlining healthcare procedures. The following are a few real-world applications of machine learning in medicine:
Medical Imaging: Medical pictures, including X-rays, MRIs, and CT scans, are analyzed using machine learning algorithms to identify anomalies, cancers, fractures, and other abnormalities with high accuracy.
Disease Diagnosis: Using patient data, symptoms, and medical history, machine learning models can help doctors diagnose conditions including cancer, diabetes, and cardiovascular illnesses.
Personalized Medicine: By evaluating genetic information, biomarkers, and patient reactions to various treatments, machine learning provides tailored treatment regimens and medication recommendations that enhance patient outcomes and minimize side effects.
Case Study: DeepMind's AI for Retinal Disease Diagnosis
An artificial intelligence (AI) system called DeepMind, a division of Alphabet Inc., the parent company of Google, was created to diagnose retinal disorders using optical coherence tomography (OCT) scans. These diseases include diabetic retinopathy and age-related macular degeneration. The AI model showed promise for early illness detection and intervention by performing on par with skilled ophthalmologists.
2. Finance:
Machine learning algorithms are employed in the finance sector for a variety of purposes, such as risk management, algorithmic trading, credit scoring, and fraud detection. Among the practical uses of machine learning in finance are:
Fraud Detection: In order to identify fraudulent behaviors in real time, such as money laundering, identity theft, and unauthorized transactions, machine learning models examine transaction data, user behavior, and past patterns.
Credit Scoring: By evaluating financial information, credit histories, and socioeconomic characteristics, machine learning algorithms evaluate the creditworthiness and risk profiles of people and companies, empowering lenders to decide on interest rates and loan approvals with confidence.
Algorithmic Trading: With the use of historical data, sentiment analysis of news, and macroeconomic indicators, machine learning models forecast stock prices, market trends, and trading patterns. This makes automated trading methods and portfolio management possible.
Case Study: JPMorgan Chase's AI for Credit Risk Assessment
For small and medium-sized enterprises, JPMorgan Chase created an AI-powered credit risk assessment system to increase the precision and effectiveness of loan rating. The AI model evaluates a variety of financial data to forecast credit risk and establish loan eligibility, including balance sheets, cash flow statements, and industry standards.
3. Ecommerce:
Machine learning is essential to the e-commerce sector because it may improve consumer experience, personalize product recommendations, optimize pricing tactics, and identify fraudulent activity. Among the practical uses of machine learning in e-commerce are:
Personalized Recommendations: To provide individualized product recommendations, boost engagement, and boost sales, machine learning algorithms examine user behavior, past purchases, and browsing habits.
Dynamic Pricing: Retailers are able to maximize income, minimize costs, and retain competitiveness by using machine learning models to improve pricing strategies depending on variables like demand, competition, and market circumstances.
Fraud Detection: By evaluating user activity, transaction data, and behavioral patterns, machine learning algorithms identify fraudulent transactions, account takeover attempts, and payment fraud, minimizing financial losses and safeguarding clients.
Case Study: Amazon's Recommendation System
Amazon's recommendation system, which makes product recommendations to users based on their browsing history, purchasing behavior, and preferences, is powered by machine learning algorithms. The recommendation system uses content-based filtering, collaborative filtering, and deep learning to provide tailored recommendations that increase customer happiness and boost sales.
Conclusion:
From a theoretical concept to a practical reality, machine learning has found widespread adoption and applications in a variety of industries. Real-world case studies highlight how machine learning can revolutionize a variety of industries, including e-commerce, healthcare, and finance. They also highlight how machine learning can spur innovation, increase productivity, and benefit both businesses and society as a whole. To fully realize the promise of this game-changing technology, practitioners, researchers, and decision-makers must keep up with the latest developments in machine learning, including best practices, emerging trends, and ethical issues. We can take on difficult problems, create new opportunities, and improve humanity's future by putting machine learning into practice.