Deep Learning vs. Machine Learning
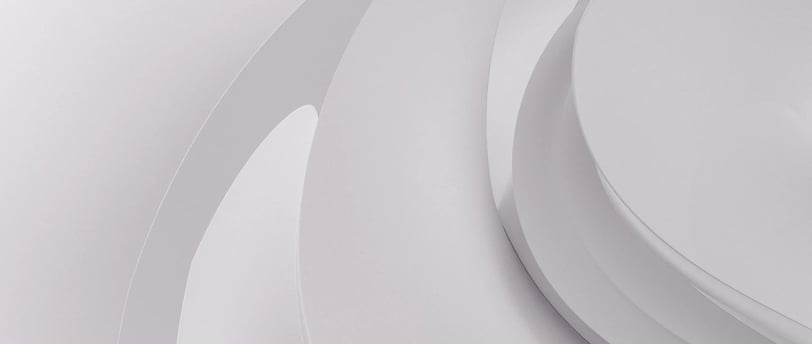
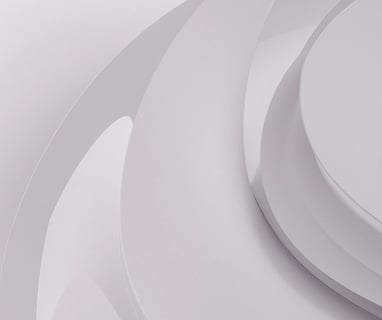
"Deep Learning vs. Machine Learning: Understanding the Difference"
Machine learning (ML) and deep learning (DL) are two of the most often debated ideas in the large field of artificial intelligence (AI). Despite the fact that they frequently serve as synonyms, they stand for different things. The goal of this blog post is to make the distinctions between the two clear and to assist you in knowing when and where to use either.
Machine Learning: A Quick Overview
Machine learning is a branch of artificial intelligence that focuses on giving computers the ability to learn from data and make judgements without being explicitly programmed. Algorithms are used by machine learning models to parse data, learn from it, and then make wise judgements based on what they have discovered.
The three main categories of machine learning are reinforcement learning, unsupervised learning, and supervised learning. The kind selected is determined by the nature of the current issue and the type of data at hand. ML underpins a wide range of everyday applications, from recommending your next Netflix movie to forecasting stock market movements.
A Deeper Dive into Deep Learning
On the other hand, deep learning is a subset of machine learning that simulates how the human brain processes information for use in decision-making. It makes use of artificial neural networks, which were created to mimic human decision-making.
Dealing with huge, unstructured data sets is where deep learning shines. It excels in fields like speech and picture recognition where it can absorb enormous volumes of data and gradually improve its predictions and judgements.
master the machine learning by taking training from softenant, one of the best machine learning training in vizag
The Important Differences
1. Data Dependencies:
While DL models require larger datasets to comprehend and learn the underlying patterns, ML models do better with fewer datasets. Due to their propensity for overfitting, deep learning models may perform poorly when trained on smaller datasets.
2. Processing Power:
ML models use less processing power and frequently operate effectively on standard CPUs. Deep learning, on the other hand, necessitates top-tier technology and GPUs because of the complexity of the computations required.
3. Interpretability:
We can easily comprehend the reasoning behind the predictions made by ML models like linear regression, decision trees, etc. This isn't the case with DL models, which are frequently referred to as 'black boxes' due to their difficulty in being understood.
4. Feature Engineering:
Choosing the best features for a model requires a lot of work in machine learning (ML). When working with high-dimensional, unstructured data, deep learning algorithms automatically learn the features, which is a big advantage.
5. Time and Resources:
Compared to a regular machine learning model, training a deep learning model often takes more time and computer resources. The vast volumes of data and intricate neural network topologies employed in deep learning are to blame for this.
Which to Use When
The decision between machine learning and deep learning mostly depends on the particular problem you're trying to solve and the kind of data you're using.
Machine learning methods can deliver efficient results with fewer processing resources for structured data or smaller datasets. Examples include credit risk scoring or customer turnover prediction.
Deep learning, on the other hand, can be more successful for huge unstructured data sets, such as picture or speech recognition applications, due to its capacity to automatically extract features and manage high dimensionality.
In conclusion, although belonging to the same AI family and working towards the same objective—making machines smarter—Machine Learning and Deep Learning have significant strengths and disadvantages. When selecting the best strategy for your AI problem, it is essential to comprehend these differences. Nevertheless, whichever path is chosen, both sectors continue to push the limits of what artificial intelligence is capable of, revolutionizing both business and everyday life.