"Advanced Techniques in Data Analytics: Moving Beyond Descriptive Statistics"
Blog post description.
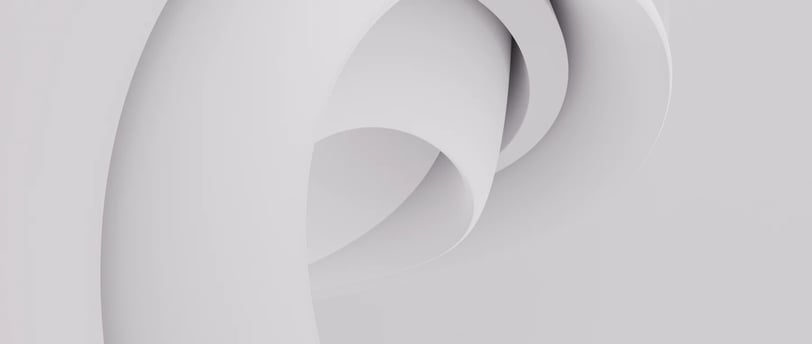
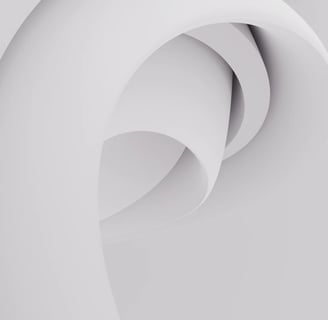
Introduction
Descriptive statistics summarize and describe the essential features of data, giving readers a basic comprehension of the data in the discipline of data analytics. Organizations must investigate advanced data analytics approaches in order to go beyond descriptive statistics and obtain deeper insights and make more educated decisions. These strategies help businesses to find hidden patterns, forecast future trends, and extract useful insights from large, complicated datasets. They do this by utilizing advanced statistical techniques, machine learning algorithms, and data mining methodologies. This thorough tutorial will cover sophisticated data analytics techniques and how to use them to address real-world issues and promote corporate success.
The Limitations of Descriptive Statistics
Although they are useful for summarizing data, descriptive statistics like mean, median, mode, and standard deviation are not very good at revealing underlying patterns, correlations, or trends. Although descriptive statistics are useful for explaining past events, they may not necessarily shed light on the reasons behind them or indicate future events that are likely to occur. Advanced data analytics approaches make use of more complex modeling and analysis tools in an effort to overcome these constraints.
Exploring Advanced Techniques
1. Predictive Analytics: Predictive analytics is the process of forecasting future events or results based on past data. Predictive analytics frequently makes use of methods like regression analysis, time series analysis, and machine learning algorithms (such as decision trees, random forests, and neural networks). Predictive analytics is a useful tool for businesses to forecast revenue, anticipate client attrition, improve marketing campaigns, and spot possible dangers or opportunities.
2. Prescriptive Analytics: Beyond forecasting future events, prescriptive analytics suggests courses of action for organizations to follow in order to accomplish their goals. In order to assist organizations in making data-driven decisions, this includes optimization approaches, simulation models, and decision support systems. Prescriptive analytics, for instance, can be applied to enhance profitability, effectively manage resources, and optimize supply chain operations.
3. Text Analytics: In order to extract sentiment and insights, text analytics analyzes unstructured text data from sources including emails, social media posts, customer reviews, and documents. Text analytics frequently makes use of topic modeling methods, sentiment analysis, and natural language processing (NLP) approaches. Businesses can utilize text analytics to track brand sentiment in real time, identify new patterns, and comprehend client comments.
4. Spatial Analytics: To find patterns and trends that are hidden in tabular data, spatial analytics analyzes geographic data and spatial relationships. Spatial analytics frequently makes use of geographic information systems (GIS), spatial regression models, and spatial clustering methods. Businesses can utilize spatial analytics to design transit routes, optimize retail placements, and examine how location-based elements affect overall business success.
5. Network Analytics: In order to pinpoint important influencers, communities, and trends, network analytics examines the behavior and structure of networks, including social, communication, and transportation networks. Commonly employed in network analytics are approaches like link prediction methods, community detection algorithms, and centrality metrics. Network analytics can help businesses find key consumers, spot fraud and other irregularities, and increase network performance.
RealWorld Applications
Let's explore some realworld applications of advanced techniques in data analytics:
Predictive maintenance in manufacturing: proactively scheduling maintenance and forecasting equipment failures with the use of predictive analytics to reduce downtime and maximize output.
Personalized recommendations in ecommerce: enhancing sales and customer satisfaction by applying machine learning algorithms to evaluate consumer behavior and preferences and provide tailored product recommendations.
Fraud detection in finance: minimizing financial losses and minimizing risks by applying anomaly detection algorithms to find unusual patterns and transactions suggestive of fraudulent conduct.
Healthcare analytics: identifying patients at risk of chronic illnesses or problems by predictive modeling, allowing for early intervention and bettering health outcomes.
Conclusion
Advanced data analytics methods give businesses strong capabilities for gaining insights, forecasting results, and making data-driven choices. Organizations may realize the full value of their data and obtain a competitive advantage in today's data-driven world by embracing predictive analytics, prescriptive analytics, text analytics, spatial analytics, and network analytics instead of just descriptive statistics. Organizations looking to prosper and develop in the digital era will need to learn sophisticated data analytics skills as the volume and complexity of data continue to rise.